Introduction
In today’s rapidly evolving world, artificial intelligence (AI) and machine learning (ML) have emerged as transformative technologies with significant implications for society and businesses. Understanding the inner workings of AI and ML is crucial to harnessing their potential and incorporating them effectively into organizational strategies. This article explores the fundamentals of AI and ML, delves into their inner workings, examines the societal implications they present, and provides insights on implementing AI within organizations.
Understanding Artificial Intelligence (AI)
Definition and Fundamentals of AI
AI refers to the simulation of human intelligence in machines, enabling them to perform tasks that typically require human intelligence machine intelligence encompasses a wide array of applications, including natural language processing, computer vision, and expert systems. Moreover, while narrow AI focuses on specific tasks, general AI aims to exhibit human-level intelligence across a broad spectrum of diverse tasks.
Machine Learning (ML) Basics
ML is a subset of AI that focuses on algorithms and statistical models that enable systems to learn from data and make predictions or decisions without explicit programming. Supervised, unsupervised, and reinforcement learning are the primary categories of ML techniques. Common ML algorithms include linear regression, decision trees, support vector machines, and deep learning algorithms.
Deep Learning and Neural Networks
Deep learning is a subset of ML that utilizes artificial neural networks with multiple layers to learn hierarchical representations of data. Neural networks are computational models inspired by the human brain and are crucial in processing complex patterns and making predictions. Popular deep learning architectures include convolutional neural networks (CNNs) for image processing and recurrent neural networks (RNNs) for sequential data analysis.
The Inner Workings of AI & ML
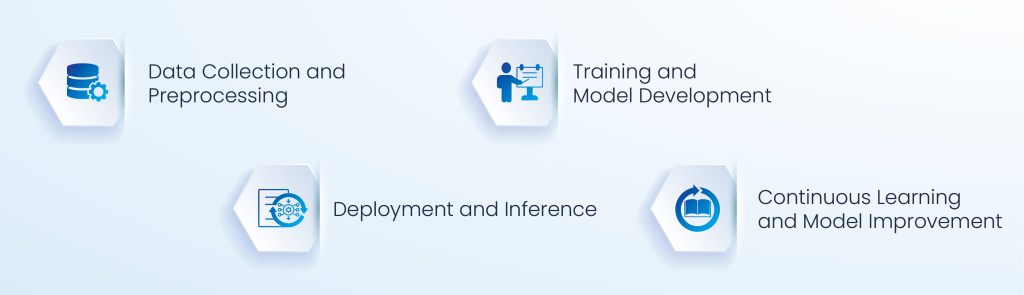
Data Collection and Preprocessing
High-quality and diverse datasets are essential for training AI and ML models. Data preprocessing techniques, including cleaning, normalization, and feature extraction, play a crucial role in ensuring data quality and compatibility for modeling purposes.
Training and Model Development
Training ML models involves feeding them with labeled data to learn patterns and optimize model parameters. Feature selection, model selection, and hyperparameter tuning play crucial roles in developing effective ML models. Validation and evaluation metrics, such as accuracy, precision, recall, and F1 score, assess the performance and generalization of trained models.
Deployment and Inference
Deploying trained models involves integrating them into production systems or platforms. Real-time inference allows models to make predictions in real-time, while batch inference processes data in batches for efficiency. Edge computing and cloud-based AI deployment provide different options for executing AI models depending on computational requirements and network constraints.
Continuous Learning and Model Improvement
Continuous learning involves retraining and updating models as new data becomes available. Techniques like transfer learning, where models leverage knowledge from pre-trained models, and ensembling, which combines predictions from multiple models, can enhance model performance.
Societal Implications of AI & ML
Ethical Considerations
AI and ML raise ethical challenges, including bias, fairness, and transparency in decision-making systems. Establishing ethical guidelines and regulations is crucial to ensure responsible development and deployment of AI systems.
Impact on Employment and Workforce
AI and ML have the potential to impact jobs and professions by automating certain tasks and augmenting human labor. Opportunities for reskilling and upskilling can mitigate job displacement and enable individuals to work alongside AI technologies.
Privacy and Security Concerns
Privacy issues arise from the collection and usage of personal data in AI and ML systems. Robust security measures and responsible data handling practices are essential to protect against potential vulnerabilities and breaches.
Implementing AI in Your Organization
Assessing Organizational Readiness
Key factors for determining organizational readiness include technological infrastructure, data availability, and employee skill sets. Leadership buy-in and cultural alignment are vital to drive successful AI adoption.
Identifying AI Opportunities
Organizations can identify potential AI use cases by analyzing existing processes, data availability, and industry trends. Examples of industries benefiting from AI adoption include healthcare, finance, manufacturing, and customer service.
Data Strategy and Infrastructure
Developing a robust data strategy involves defining data requirements, ensuring data quality, and establishing data governance frameworks. Adequate data infrastructure and storage solutions are necessary to handle the volume and velocity of data required for AI implementation.
Building an AI Team
Building a capable AI team requires a diverse set of roles, including data scientists, machine learning engineers, and domain experts. Hiring and upskilling employees for AI-related roles can help organizations develop the necessary expertise in-house.
Overcoming Challenges and Risks
Common challenges in AI implementation include data quality issues, lack of expertise, and resistance to change. Strategies for managing risks include conducting pilot projects, fostering a culture of experimentation, and ensuring ongoing collaboration between business and technical teams.
Conclusion
In conclusion, understanding the inner workings of AI and ML is essential for individuals and organizations aiming to harness the potential of these technologies. We explored the fundamentals of AI and ML, delved into their inner workings, and discussed the societal implications they present. Additionally, we provided insights on implementing AI within organizations, addressing challenges, and fostering responsible practices. By embracing AI while considering ethical considerations, organizations can unlock new possibilities and drive innovation in the digital era.